You are looking for information, articles, knowledge about the topic nail salons open on sunday near me 스파크 딥 러닝 on Google, you do not find the information you need! Here are the best content compiled and compiled by the https://toplist.pilgrimjournalist.com team, along with other related topics such as: 스파크 딥 러닝 스파크 머신러닝, 스파크 완벽 가이드 PDF, Pyspark ML, Spark MLlib 예제, Spark 회귀분석, Spark .sql 한글, 아파치 스파크, Pyspark Deep learning
Table of Contents
Spark을 이용한 Deeplearning — 씨앤텍시스템즈 기술블로그
- Article author: cntechsystems.tistory.com
- Reviews from users: 44762
Ratings
- Top rated: 3.3
- Lowest rated: 1
- Summary of article content: Articles about Spark을 이용한 Deeplearning — 씨앤텍시스템즈 기술블로그 딥러닝에 대해서 간략하게 알아보자면 머신러닝의 한 분야로써, 뇌의 신경망 구조를 모방한 알고리즘으로 인공신경망 알고리즘을 활용하고 있습니다. 기존 … …
- Most searched keywords: Whether you are looking for Spark을 이용한 Deeplearning — 씨앤텍시스템즈 기술블로그 딥러닝에 대해서 간략하게 알아보자면 머신러닝의 한 분야로써, 뇌의 신경망 구조를 모방한 알고리즘으로 인공신경망 알고리즘을 활용하고 있습니다. 기존 … 안녕하세요 씨앤텍시스템즈입니다. 본 포스팅에서는 Spark를 이용하여 Deeplearning을 수행하는 방법을 알아봅니다. 이전 포스팅을 통해서 스파크에 대한 개요(https://cntechsystems.tistory.com/62?category=7..
- Table of Contents:
블로그 메뉴
공지사항
인기 글
최근 글
최근 댓글
태그
티스토리
푸터 메뉴
정보
티스토리툴바
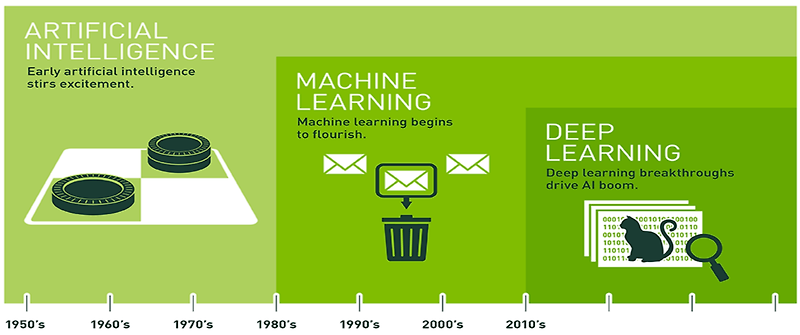
Machine Learning with Spark (Spark로 머신러닝하기) – Mk’s Blog
- Article author: moons08.github.io
- Reviews from users: 25414
Ratings
- Top rated: 3.2
- Lowest rated: 1
- Summary of article content: Articles about Machine Learning with Spark (Spark로 머신러닝하기) – Mk’s Blog 스파크의 분산처리 능력을 머신러닝에 사용할 수 있습니다. 간단한 자연어 처리 예제와 함께 알아 … 무려 딥러닝도 스파크로 돌릴 수도 있습니다! …
- Most searched keywords: Whether you are looking for Machine Learning with Spark (Spark로 머신러닝하기) – Mk’s Blog 스파크의 분산처리 능력을 머신러닝에 사용할 수 있습니다. 간단한 자연어 처리 예제와 함께 알아 … 무려 딥러닝도 스파크로 돌릴 수도 있습니다! 스파크의 분산처리 능력을 머신러닝에 사용할 수 있습니다. 간단한 자연어 처리 예제와 함께 알아보겠습니다. 비교적 쉽게 접할 수 있는 scikit-learn, R, tensorflow와 달리 SparkML은 흔치 않지만, 나름대로의 장점이 있습니다.
- Table of Contents:
Skip links
Intro
Classification Pipeline
Outro
2022년 상반기 회고 (확장하는 팀과 개인 좋은 동료와 성장 그리고 스트레스 관리)
어느새 4년차가 된 데이터 엔지니어의 2021년 회고
Slack SDK로 메세지 보내기 (with Airflow)
SDK를 쓰는 이유와 aws boto3
[논문]스파크 기반 딥 러닝 분산 프레임워크 성능 비교 분석
- Article author: scienceon.kisti.re.kr
- Reviews from users: 44652
Ratings
- Top rated: 4.8
- Lowest rated: 1
- Summary of article content: Articles about [논문]스파크 기반 딥 러닝 분산 프레임워크 성능 비교 분석 딥 러닝(Deep learning)은 기존 인공 신경망 내 계층 수를 증가시킴과 동시에 효과적인 학습 방법론을 제시함으로써 객체/음성 인식 및 자연어 처리 등 고수준 문제 … …
- Most searched keywords: Whether you are looking for [논문]스파크 기반 딥 러닝 분산 프레임워크 성능 비교 분석 딥 러닝(Deep learning)은 기존 인공 신경망 내 계층 수를 증가시킴과 동시에 효과적인 학습 방법론을 제시함으로써 객체/음성 인식 및 자연어 처리 등 고수준 문제 … 딥 러닝, 스파크, 분산 컴퓨팅, 클러스터 컴퓨팅딥 러닝(Deep learning)은 기존 인공 신경망 내 계층 수를 증가시킴과 동시에 효과적인 학습 방법론을 제시함으로써 객체/음성 인식 및 자연어 처리 등 고수준 문제 해결에 있어 괄목할만한 성과를 보이고 있다. 그러나 학습에 필요한 시간과 리소스가 크다는 한계를 지니고 있어, 이를 줄이기 위한 연구가 활발히 진행되고 있다. 본 연구에서는 아파치 스파크 기반 클러스터 컴퓨팅 프레임워크 상에서 딥 러닝을 분산화하는 두 가지 툴(DeepSpark, SparkNet)의 성능을 학습 정확도와 속도 측면에서 측정하고 분석하였다. CIFAR-10/CIFAR-100 데이터를 사용한 실험에서 SparkNet은 학습 과정의 정확도 변동 폭이 적은 반면 DeepSpark는 학습 초기 정확도는 변동 폭이 크지만 점차 변동 폭이 줄어들면서 SparkNet 대비 약 15% 높은 정확도를 보였고, 조건에 따라 단일 머신보다도 높은 정확도로 보다 빠르게 수렴하는 양상을 확인할 수 있었다.
- Table of Contents:
스파크 기반 딥 러닝 분산 프레임워크 성능 비교 분석
인용
A Comparative Performance Analysis of Spark-Based Distributed Deep-Learning Frameworks
상세정보조회
원문조회
![[논문]스파크 기반 딥 러닝 분산 프레임워크 성능 비교 분석](https://scienceon.kisti.re.kr/images/usr/scienceon_og.png)
분석 및 머신 러닝용 Apache Spark 3.0 | NVIDIA
- Article author: www.nvidia.com
- Reviews from users: 35718
Ratings
- Top rated: 3.6
- Lowest rated: 1
- Summary of article content: Articles about 분석 및 머신 러닝용 Apache Spark 3.0 | NVIDIA GPU 가속 Apache Spark 데이터 분석, 머신 러닝, 딥 러닝 파이프라인용. Apache Spark 3 데이터 사이언스 파이프라인을 코드 변경 없이 GPU 가속화하여 인프라 비용을 … …
- Most searched keywords: Whether you are looking for 분석 및 머신 러닝용 Apache Spark 3.0 | NVIDIA GPU 가속 Apache Spark 데이터 분석, 머신 러닝, 딥 러닝 파이프라인용. Apache Spark 3 데이터 사이언스 파이프라인을 코드 변경 없이 GPU 가속화하여 인프라 비용을 … Apache Spark3.0, NVIDIA Apache Spark 3.0, ETL, data pipelines, Machine Learning pipelines, Analytics pipelinesApache Spark 3.0은 일련의 사용하기 간편한 ETL용 API, 머신 러닝 및 다양한 소스의 방대한 데이터세트에 대해 강력한 처리 성능으로 도출한 그래프를 제공합니다.
- Table of Contents:
Apache Spark를 선택해야 하는 이유
NVIDIA GPU와 함께하는 Spark의 주요 혜택
Spark 3 혁신
Spark의 가속 분석 및 AI
GPU 가속 Spark 시작하기
Clouddera와 NVIDIA의 통합으로 데이터 기반 인사이트를 활용하여 미션 크리티컬한 활용 사례를 향상시킬 수 있습니다 저희는 현재 이 통합을 구현하고 있으며 이미 데이터 엔지니어링 및 데이터 사이언스 워크플로우 비용의 절반으로 10배 이상의 속도 향상을 경험하고 있습니다
NVIDIA는 CPU에서 Spark를 실행하는 것에 비해 NVIDIA 가속 Spark 3을 실행함으로써 엄청나게 더 빨라진 성능을 경험하고 있습니다 이러한 획기적인 GPU 성능 혜택으로 당사의 전체 Adobe Experience Cloud 앱 제품군에서 AI 기반 기능에 대한 완전히 새로운 가능성이 열리고 있습니다
NVIDIA와의 지속적인 협력으로 Databricks와 Apache Spark 3에 대한 RAPIDS 최적화 관련 성능이 향상되어 Adobe 등의 공동 고객이 이점을 누리고 있습니다 이러한 기여 덕분에 데이터 파이프라인 모델 트레이닝 및 채점이 더욱 빨라지게 되어 데이터 엔지니어와 데이터 사이언티스트 커뮤니티에서 더 많은 돌파구와 인사이트를 바로 얻을 수 있습니다
Clouddera와 NVIDIA의 통합으로 데이터 기반 인사이트를 활용하여 미션 크리티컬한 활용 사례를 향상시킬 수 있습니다 저희는 현재 이 통합을 구현하고 있으며 이미 데이터 엔지니어링 및 데이터 사이언스 워크플로우 비용의 절반으로 10배 이상의 속도 향상을 경험하고 있습니다
NVIDIA는 CPU에서 Spark를 실행하는 것에 비해 NVIDIA 가속 Spark 3을 실행함으로써 엄청나게 더 빨라진 성능을 경험하고 있습니다 이러한 획기적인 GPU 성능 혜택으로 당사의 전체 Adobe Experience Cloud 앱 제품군에서 AI 기반 기능에 대한 완전히 새로운 가능성이 열리고 있습니다
NVIDIA와의 지속적인 협력으로 Databricks와 Apache Spark 3에 대한 RAPIDS 최적화 관련 성능이 향상되어 Adobe 등의 공동 고객이 이점을 누리고 있습니다 이러한 기여 덕분에 데이터 파이프라인 모델 트레이닝 및 채점이 더욱 빨라지게 되어 데이터 엔지니어와 데이터 사이언티스트 커뮤니티에서 더 많은 돌파구와 인사이트를 바로 얻을 수 있습니다
Clouddera와 NVIDIA의 통합으로 데이터 기반 인사이트를 활용하여 미션 크리티컬한 활용 사례를 향상시킬 수 있습니다 저희는 현재 이 통합을 구현하고 있으며 이미 데이터 엔지니어링 및 데이터 사이언스 워크플로우 비용의 절반으로 10배 이상의 속도 향상을 경험하고 있습니다
NVIDIA는 CPU에서 Spark를 실행하는 것에 비해 NVIDIA 가속 Spark 3을 실행함으로써 엄청나게 더 빨라진 성능을 경험하고 있습니다 이러한 획기적인 GPU 성능 혜택으로 당사의 전체 Adobe Experience Cloud 앱 제품군에서 AI 기반 기능에 대한 완전히 새로운 가능성이 열리고 있습니다
NVIDIA와의 지속적인 협력으로 Databricks와 Apache Spark 3에 대한 RAPIDS 최적화 관련 성능이 향상되어 Adobe 등의 공동 고객이 이점을 누리고 있습니다 이러한 기여 덕분에 데이터 파이프라인 모델 트레이닝 및 채점이 더욱 빨라지게 되어 데이터 엔지니어와 데이터 사이언티스트 커뮤니티에서 더 많은 돌파구와 인사이트를 바로 얻을 수 있습니다
Spark 전문가에게 문의하기
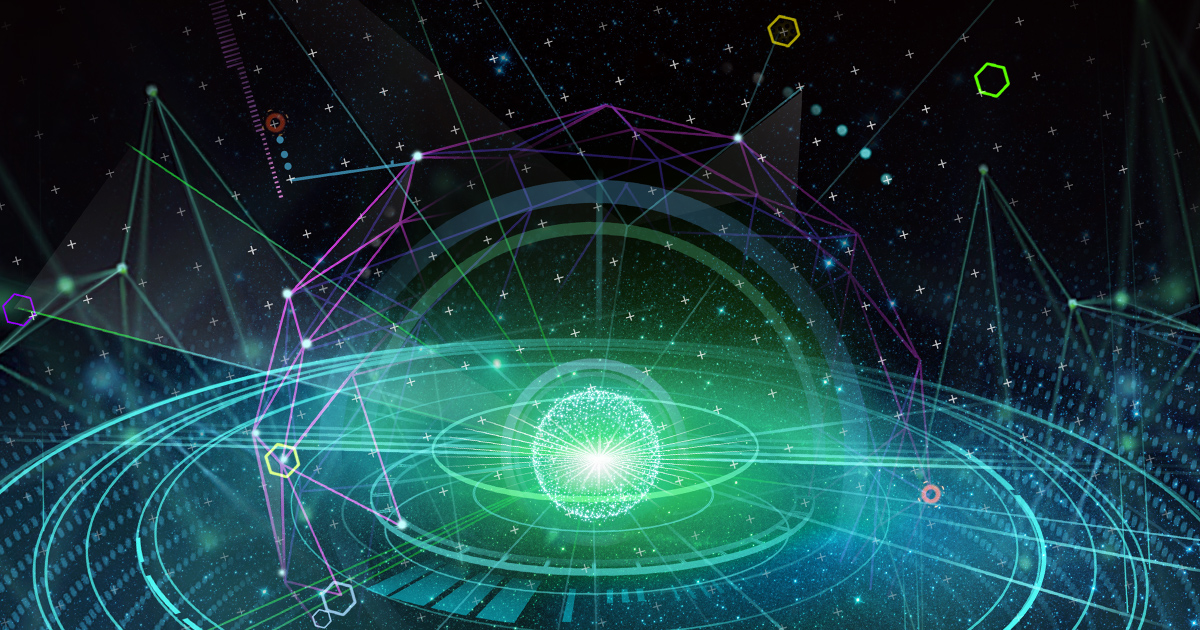
GitHub – databricks/spark-deep-learning: Deep Learning Pipelines for Apache Spark
- Article author: github.com
- Reviews from users: 47219
Ratings
- Top rated: 4.1
- Lowest rated: 1
- Summary of article content: Articles about GitHub – databricks/spark-deep-learning: Deep Learning Pipelines for Apache Spark Deep Learning Pipelines for Apache Spark. Contribute to databricks/spark-deep-learning development by creating an account on GitHub. …
- Most searched keywords: Whether you are looking for GitHub – databricks/spark-deep-learning: Deep Learning Pipelines for Apache Spark Deep Learning Pipelines for Apache Spark. Contribute to databricks/spark-deep-learning development by creating an account on GitHub. Deep Learning Pipelines for Apache Spark. Contribute to databricks/spark-deep-learning development by creating an account on GitHub.
- Table of Contents:
Latest commit
Git stats
Files
READMEmd
API Documentation
Releases
License
About
Releases
9
Packages 0
Contributors 17
Languages
Footer
GitHub – databricks/spark-deep-learning: Deep Learning Pipelines for Apache Spark
- Article author: towardsdatascience.com
- Reviews from users: 2301
Ratings
- Top rated: 3.2
- Lowest rated: 1
- Summary of article content: Articles about GitHub – databricks/spark-deep-learning: Deep Learning Pipelines for Apache Spark Deep Learning Pipelines is an open source library created by Databricks that proves high-level APIs for scalable deep learning in Python with … …
- Most searched keywords: Whether you are looking for GitHub – databricks/spark-deep-learning: Deep Learning Pipelines for Apache Spark Deep Learning Pipelines is an open source library created by Databricks that proves high-level APIs for scalable deep learning in Python with … Deep Learning Pipelines for Apache Spark. Contribute to databricks/spark-deep-learning development by creating an account on GitHub.
- Table of Contents:
Latest commit
Git stats
Files
READMEmd
API Documentation
Releases
License
About
Releases
9
Packages 0
Contributors 17
Languages
Footer
스파크 기반 딥 러닝 분산 프레임워크 성능 비교 분석
- Article author: dspace.kci.go.kr
- Reviews from users: 21691
Ratings
- Top rated: 4.8
- Lowest rated: 1
- Summary of article content: Articles about 스파크 기반 딥 러닝 분산 프레임워크 성능 비교 분석 딥 러닝(Deep learning)은 기존 인공 신경망 내 계층 수를 증가시킴과 동시에 효과적인 학습방법론을 제시함으로써 객체/음성 인식 및 자연어 처리 등 고수준 문제 … …
- Most searched keywords: Whether you are looking for 스파크 기반 딥 러닝 분산 프레임워크 성능 비교 분석 딥 러닝(Deep learning)은 기존 인공 신경망 내 계층 수를 증가시킴과 동시에 효과적인 학습방법론을 제시함으로써 객체/음성 인식 및 자연어 처리 등 고수준 문제 … A Comparative Performance Analysis of Spark-Based Distributed Deep-Learning Frameworks – deep learning;Apache Spark;Caffe;parallel computing;cluster computing
- Table of Contents:
초록
열기닫기 버튼
키워드열기닫기 버튼
인용현황
KCI에서 이 논문을 인용한 논문의 수는 2건입니다
열기닫기 버튼
참고문헌(16)
열기닫기 버튼
2020년 이후 발행 논문의 참고문헌은 현재 구축 중입니다

See more articles in the same category here: Toplist.pilgrimjournalist.com/blog.
Spark을 이용한 Deeplearning
안녕하세요 씨앤텍시스템즈입니다.
본 포스팅에서는 Spark를 이용하여 Deeplearning을 수행하는 방법을 알아봅니다.
이전 포스팅을 통해서 스파크에 대한 개요(https://cntechsystems.tistory.com/62?category=786008)와 데이터 처리(https://cntechsystems.tistory.com/82?category=786008)방법을 알아보았습니다. 빅데이터 기술분야에서 막강한 기능을 제공하는 스파크는 최근 인공지능 발전의 마중물을 역할을 하게 된 딥러닝 마저도 지원될거라 생각이 되지만, 아직 정식으로 지원되는 기술은 아닙니다.
http://blogs.nvidia.co.kr/2016/08/03/difference_ai_learning_machinelearning
딥러닝에 대해서 간략하게 알아보자면 머신러닝의 한 분야로써, 뇌의 신경망 구조를 모방한 알고리즘으로 인공신경망 알고리즘을 활용하고 있습니다. 기존 머신러닝 학습 또는 모델생성 과정과는 조금의 차이가 있는데, 기존 머신러닝에서는 사람이 Feature, 특징 등을 지정하고 모델에서 이미지 분류 수행 또는 학습하였지만 딥러닝에서는 모델 자체에서 Feature와 특징, 분류까지 모두 수행한다는 점에서 다른점이 있습니다.
스파크에서 머신러닝 알고리즘(Regression, Classification, Clustering, NLP)은 이미 다양하게 제공하고 있지만 인-메모리 지원이라는 특징 때문에 많은 양의 데이터를 학습하고 깊은 레이어를 쌓아 많은 연산을 필요로 하는 딥러닝에서는 사실 GPU를 이용한 방식으로 자리를 잡았기 때문입니다. 하지만, 스파크를 이용하여 딥러닝을 수행하고 지원하고자 하는 많은 라이브러리와 시도들이 있었습니다.
SparkDL(Databricks)
TensorflowOnSpark(Yahoo)
BigDL(Intel)
H2O
스파크에서는 딥러닝 Pipeline을 제공하며 이를 이용하여 딥 러닝 라이브러리와 분류기를 호출할 수 있으며 텐서플로우나 케라스 모델에도 적용할 수 있습니다.
H2O
H2O는 오픈소스 머신러닝 플랫폼이며, 딥러닝 / 일반 선형 모델 / 랜덤 포레스트 / 나이브 베이즈 / 군집화 / 그레이디언트 부스팅 머신 / 서포트 벡터 머신 등 다양한 머신러닝 알고리즘을 제공하고 특히 스파크에서 제공되지 않는 딥러닝 알고리즘 등을 제공한다. H2O는 R과 Python에서도 사용 가능합니다.
https://www.h2o.ai/
H2O에서는 스파클링 워터(SPARKLING WATER)라는 스파크 패키지 형태로 구현된 통합레이어를 제공합니다. 아래와 아키텍처 그림처럼, 스파크 클러스터의 드라이버 노드와 익스큐터를 H2O의 H2O Context와 H2O노드를 통합하여 데이터를 교환하고 다양한 머신러닝 알고리즘을 수행할 수 있습니다.
https://www.h2o.ai/products/h2o-sparkling-water/
SparklingWater 수행 예제
In [1]: from pysparkling import * import h2o In [2]: hc = H2OContext.getOrCreate(spark) Connecting to H2O server at http://192.168.56.101:54321 … successful. H2O cluster uptime: 14 secs H2O cluster timezone: Asia/Seoul H2O data parsing timezone: UTC H2O cluster version: 3.28.0.1 H2O cluster version age: 1 month and 20 days H2O cluster name: sparkling-water-carbig_local-1580951039227 H2O cluster total nodes: 1 H2O cluster free memory: 808 Mb H2O cluster total cores: 2 H2O cluster allowed cores: 2 H2O cluster status: accepting new members, healthy H2O connection url: http://192.168.56.101:54321 H2O connection proxy: None H2O internal security: False H2O API Extensions: XGBoost, Algos, Amazon S3, AutoML, Core V3, TargetEncoder, Core V4 Python version: 3.7.4 final Sparkling Water Context: * Sparkling Water Version: 3.28.0.1-1-2.4 * H2O name: sparkling-water-carbig_local-1580951039227 * cluster size: 1 * list of used nodes: (executorId, host, port) ———————— (driver,192.168.56.101,54321) ———————— Open H2O Flow in browser: http://192.168.56.101:54321 (CMD + click in Mac OSX) In [3]: housingDF = spark.read.csv(“data/housing.data”, inferSchema=True, header=True) In [4]: housingDF.printSchema() root |– 0.00632: double (nullable = true) |– 18.00: double (nullable = true) |– 2.310: double (nullable = true) |– 0: double (nullable = true) |– 0.5380: double (nullable = true) |– 6.5750: double (nullable = true) |– 65.20: double (nullable = true) |– 4.0900: double (nullable = true) |– 1: double (nullable = true) |– 296.0: double (nullable = true) |– 15.30: double (nullable = true) |– 396.90: double (nullable = true) |– 4.98: double (nullable = true) |– 24.00: double (nullable = true) In [5]: housingDF.show(5) +——–+——-+——–+—+——–+——–+——-+——–+—-+——-+——-+——-+——-+——-+ | 0.00632| 18.00| 2.310| 0| 0.5380| 6.5750| 65.20| 4.0900| 1| 296.0| 15.30| 396.90| 4.98| 24.00| +——–+——-+——–+—+——–+——–+——-+——–+—-+——-+——-+——-+——-+——-+ | 0.02731| 0.0| 7.07|0.0| 0.469| 6.421| 78.9| 4.9671| 2.0| 242.0| 17.8| 396.9| 9.14| 21.6| | 0.02729| 0.0| 7.07|0.0| 0.469| 7.185| 61.1| 4.9671| 2.0| 242.0| 17.8| 392.83| 4.03| 34.7| | 0.03237| 0.0| 2.18|0.0| 0.458| 6.998| 45.8| 6.0622| 3.0| 222.0| 18.7| 394.63| 2.94| 33.4| | 0.06905| 0.0| 2.18|0.0| 0.458| 7.147| 54.2| 6.0622| 3.0| 222.0| 18.7| 396.9| 5.33| 36.2| | 0.02985| 0.0| 2.18|0.0| 0.458| 6.43| 58.7| 6.0622| 3.0| 222.0| 18.7| 394.12| 5.21| 28.7| +——–+——-+——–+—+——–+——–+——-+——–+—-+——-+——-+——-+——-+——-+ only showing top 5 rows In [6]: from pyspark.sql import Row from pyspark.sql.types import StructField, StructType, DoubleType In [7]: housingSchema = StructType([ StructField(‘crim’, DoubleType(), True), StructField(‘zn’, DoubleType(), True), StructField(‘indus’, DoubleType(), True), StructField(‘chas’, DoubleType(), True), StructField(‘nox’, DoubleType(), True), StructField(‘rm’, DoubleType(), True), StructField(‘age’, DoubleType(), True), StructField(‘dis’, DoubleType(), True), StructField(‘rad’, DoubleType(), True), StructField(‘tax’, DoubleType(), True), StructField(‘ptratio’, DoubleType(), True), StructField(‘b’, DoubleType(), True), StructField(‘lstat’, DoubleType(), True), StructField(‘medv’, DoubleType(), True), ]) In [8]: housingDF = spark.read.csv(“data/housing.data”, inferSchema=True, schema=housingSchema) In [9]: housingDF.show(5) +——-+—-+—–+—-+—–+—–+—-+——+—+—–+——-+——+—–+—-+ | crim| zn|indus|chas| nox| rm| age| dis|rad| tax|ptratio| b|lstat|medv| +——-+—-+—–+—-+—–+—–+—-+——+—+—–+——-+——+—–+—-+ |0.00632|18.0| 2.31| 0.0|0.538|6.575|65.2| 4.09|1.0|296.0| 15.3| 396.9| 4.98|24.0| |0.02731| 0.0| 7.07| 0.0|0.469|6.421|78.9|4.9671|2.0|242.0| 17.8| 396.9| 9.14|21.6| |0.02729| 0.0| 7.07| 0.0|0.469|7.185|61.1|4.9671|2.0|242.0| 17.8|392.83| 4.03|34.7| |0.03237| 0.0| 2.18| 0.0|0.458|6.998|45.8|6.0622|3.0|222.0| 18.7|394.63| 2.94|33.4| |0.06905| 0.0| 2.18| 0.0|0.458|7.147|54.2|6.0622|3.0|222.0| 18.7| 396.9| 5.33|36.2| +——-+—-+—–+—-+—–+—–+—-+——+—+—–+——-+——+—–+—-+ only showing top 5 rows In [10]: housingDFh20 = hc.as_h2o_frame(housingDF, ‘housing’) #spark에서 만든 데이터 프레임을 h20에서 쓸 수 있도록 In [11]: splitDF = housingDFh20.split_frame([0.75,0.24]) #합이 1이 안되게 In [12]: train = splitDF[0] In [13]: train.frame_id = ‘housing_train’ In [14]: test = splitDF[1] test.frame_id = ‘housing_test’ In [15]: from h2o.estimators.deeplearning import H2ODeepLearningEstimator In [29]: m = H2ODeepLearningEstimator(hidden=[200, 200, 200], epochs=800, activation=’rectifierwithdropout’,\ hidden_dropout_ratios=[0.4,0.2,0.4]) In [30]: m.train(x=train.names[:-1], y=train.names[13], training_frame=train, validation_frame=test) deeplearning Model Build progress: |██████████████████████████████████████| 100% In [28]: m.show() Model Details ============= H2ODeepLearningEstimator : Deep Learning Model Key: DeepLearning_model_python_1580951124328_4 Status of Neuron Layers: predicting medv, regression, gaussian distribution, Quadratic loss, 326,801 weights/biases, 3.8 MB, 228,600 training samples, mini-batch size 1 layer units type dropout l1 l2 mean_rate rate_rms momentum mean_weight weight_rms mean_bias bias_rms 0 1 13 Input 0 1 2 400 RectifierDropout 40 0 0 0.0298622 0.0174399 0 -0.0014201 0.13315 0.341532 0.0858229 2 3 400 RectifierDropout 20 0 0 0.0760471 0.0467215 0 -0.0191901 0.0649896 0.934475 0.0971478 3 4 400 RectifierDropout 40 0 0 0.0570879 0.0641115 0 -0.00851255 0.0545392 0.940617 0.0345877 4 5 1 Linear 0 0 0.00146979 0.000868104 0 0.0024622 0.0399774 -0.117518 1.09713e-154 ModelMetricsRegression: deeplearning ** Reported on train data. ** MSE: 4.491494519981133 RMSE: 2.1193146344941645 MAE: 1.5742916415136954 RMSLE: 0.12387445579540285 Mean Residual Deviance: 4.491494519981133 ModelMetricsRegression: deeplearning ** Reported on validation data. ** MSE: 8.45884198732803 RMSE: 2.9084088411583457 MAE: 2.234092485453941 RMSLE: 0.15526005315606428 Mean Residual Deviance: 8.45884198732803 Scoring History: timestamp duration training_speed epochs iterations samples training_rmse training_deviance training_mae training_r2 validation_rmse validation_deviance validation_mae validation_r2 0 2020-02-06 10:48:15 0.000 sec None 0.0 0 0.0 NaN NaN NaN NaN NaN NaN NaN NaN 1 2020-02-06 10:48:19 4.595 sec 865 obs/sec 10.0 1 3810.0 4.306655 18.547280 2.803999 0.775459 4.799666 23.036791 2.904183 0.742674 2 2020-02-06 10:48:26 10.973 sec 1071 obs/sec 30.0 3 11430.0 3.785238 14.328024 2.507152 0.826539 4.053157 16.428082 2.620617 0.816495 3 2020-02-06 10:48:31 16.456 sec 1187 obs/sec 50.0 5 19050.0 3.643007 13.271497 2.679611 0.839330 3.850721 14.828051 2.911060 0.834368 4 2020-02-06 10:48:36 21.586 sec 1267 obs/sec 70.0 7 26670.0 3.194291 10.203496 2.265252 0.876472 3.363074 11.310266 2.527003 0.873662 5 2020-02-06 10:48:43 28.481 sec 1368 obs/sec 100.0 10 38100.0 3.270587 10.696737 2.363280 0.870501 3.257141 10.608968 2.478348 0.881496 6 2020-02-06 10:48:49 34.858 sec 1452 obs/sec 130.0 13 49530.0 3.226082 10.407603 2.287425 0.874001 3.468513 12.030583 2.647730 0.865616 7 2020-02-06 10:48:55 40.821 sec 1525 obs/sec 160.0 16 60960.0 3.046344 9.280211 2.163496 0.887650 3.427086 11.744918 2.555101 0.868807 8 2020-02-06 10:49:01 46.777 sec 1581 obs/sec 190.0 19 72390.0 2.877202 8.278294 2.066885 0.899780 3.116319 9.711442 2.432577 0.891521 9 2020-02-06 10:49:07 52.413 sec 1634 obs/sec 220.0 22 83820.0 3.049112 9.297085 2.234404 0.887446 3.379012 11.417720 2.578131 0.872462 10 2020-02-06 10:49:13 58.579 sec 1661 obs/sec 250.0 25 95250.0 2.830604 8.012319 2.068955 0.903000 3.150313 9.924469 2.421001 0.889142 11 2020-02-06 10:49:19 1 min 4.491 sec 1690 obs/sec 280.0 28 106680.0 2.454265 6.023416 1.793329 0.927078 2.935127 8.614973 2.173127 0.903769 12 2020-02-06 10:49:24 1 min 9.860 sec 1727 obs/sec 310.0 31 118110.0 2.589645 6.706263 1.883426 0.918811 3.270033 10.693118 2.472490 0.880556 13 2020-02-06 10:49:30 1 min 14.980 sec 1765 obs/sec 340.0 34 129540.0 2.429125 5.900646 1.783728 0.928564 3.089552 9.545335 2.389783 0.893377 14 2020-02-06 10:49:35 1 min 20.108 sec 1798 obs/sec 370.0 37 140970.0 2.393984 5.731161 1.776052 0.930616 3.104331 9.636872 2.396955 0.892354 15 2020-02-06 10:49:41 1 min 26.759 sec 1839 obs/sec 410.0 41 156210.0 2.119315 4.491495 1.574292 0.945624 2.908409 8.458842 2.234092 0.905513 16 2020-02-06 10:49:46 1 min 31.788 sec 1866 obs/sec 440.0 44 167640.0 2.072595 4.295649 1.529558 0.947995 3.003650 9.021914 2.272905 0.899223 17 2020-02-06 10:49:53 1 min 38.342 sec 1899 obs/sec 480.0 48 182880.0 1.955997 3.825923 1.421920 0.953682 3.003762 9.022586 2.243523 0.899216 18 2020-02-06 10:49:59 1 min 44.825 sec 1931 obs/sec 520.0 52 198120.0 1.890754 3.574952 1.358395 0.956720 2.986179 8.917266 2.229141 0.900392 19 2020-02-06 10:50:06 1 min 51.213 sec 1959 obs/sec 560.0 56 213360.0 1.830215 3.349685 1.327494 0.959447 2.927398 8.569658 2.192749 0.904275 See the whole table with table.as_data_frame() Variable Importances: variable relative_importance scaled_importance percentage 0 lstat 1.000000 1.000000 0.119270 1 rm 0.863339 0.863339 0.102971 2 dis 0.812267 0.812267 0.096879 3 nox 0.794105 0.794105 0.094713 4 age 0.738908 0.738908 0.088130 5 tax 0.619250 0.619250 0.073858 6 crim 0.602778 0.602778 0.071893 7 ptratio 0.567626 0.567626 0.067701 8 rad 0.562254 0.562254 0.067060 9 b 0.560138 0.560138 0.066808 10 indus 0.523724 0.523724 0.062465 11 zn 0.392638 0.392638 0.046830 12 chas 0.347298 0.347298 0.041422 In [ ]: In [ ]:
728×90
Machine Learning with Spark (Spark로 머신러닝하기)
import org.apache.spark.sql.functions._ import org.apache.spark.sql.Encoders case class GameReview ( title : String , user : String , score : Int , _review : String , _corrupt_record : String ) var df = spark . read . option ( “header” , “true” ) . option ( “inferSchema” , “true” ) . option ( “multiLine” , true ) . option ( “escape” , “\”” ) . schema ( Encoders . product [ GameReview ]. schema ) . csv ( “/data/user_detail.csv” ) df = df . withColumn ( “id” , monotonically_increasing_id ()) . withColumn ( “target” , expr ( “”” case when score > 8 then ‘good’ when score < 5 then 'bad' else 'soso' end """ )). where ( "target != 'soso'" ) df . createOrReplaceTempView ( "df" ) val Array ( train , test ) = df . randomSplit ( Array ( 0.8 , 0.2 ), seed = 543 ) // 학습용, 테스트용으로 데이터를 나눴습니다.
databricks/spark-deep-learning: Deep Learning Pipelines for Apache Spark
Deep Learning Pipelines for Apache Spark
The repo only contains HorovodRunner code for local CI and API docs. To use HorovodRunner for distributed training, please use Databricks Runtime for Machine Learning, Visit databricks doc HorovodRunner: distributed deep learning with Horovod for details.
To use the previous release that contains Spark Deep Learning Pipelines API, please go to Spark Packages page.
API Documentation
class sparkdl.HorovodRunner(*, np, driver_log_verbosity=’all’)
Bases: object
HorovodRunner runs distributed deep learning training jobs using Horovod.
On Databricks Runtime 5.0 ML and above, it launches the Horovod job as a distributed Spark job. It makes running Horovod easy on Databricks by managing the cluster setup and integrating with Spark. Check out Databricks documentation to view end-to-end examples and performance tuning tips.
The open-source version only runs the job locally inside the same Python process, which is for local development only.
NOTE: Horovod is a distributed training framework developed by Uber.
Parameters np – number of parallel processes to use for the Horovod job. This argument only takes effect on Databricks Runtime 5.0 ML and above. It is ignored in the open-source version. On Databricks, each process will take an available task slot, which maps to a GPU on a GPU cluster or a CPU core on a CPU cluster. Accepted values are: If <0, this will spawn -np subprocesses on the driver node to run Horovod locally. Training stdout and stderr messages go to the notebook cell output, and are also available in driver logs in case the cell output is truncated. This is useful for debugging and we recommend testing your code under this mode first. However, be careful of heavy use of the Spark driver on a shared Databricks cluster. Note that np < -1 is only supported on Databricks Runtime 5.5 ML and above. If >0, this will launch a Spark job with np tasks starting all together and run the Horovod job on the task nodes. It will wait until np task slots are available to launch the job. If np is greater than the total number of task slots on the cluster, the job will fail. As of Databricks Runtime 5.4 ML, training stdout and stderr messages go to the notebook cell output. In the event that the cell output is truncated, full logs are available in stderr stream of task 0 under the 2nd spark job started by HorovodRunner, which you can find in the Spark UI. If 0, this will use all task slots on the cluster to launch the job. .. warning:: Setting np=0 is deprecated and it will be removed in the next major Databricks Runtime release. Choosing np based on the total task slots at runtime is unreliable due to dynamic executor registration. Please set the number of parallel processes you need explicitly. np – driver_log_verbosity: This argument is only available on Databricks Runtime.
Runs a Horovod training job invoking main(**kwargs).
The open-source version only invokes main(**kwargs) inside the same Python process. On Databricks Runtime 5.0 ML and above, it will launch the Horovod job based on the documented behavior of np. Both the main function and the keyword arguments are serialized using cloudpickle and distributed to cluster workers.
Parameters main – a Python function that contains the Horovod training code. The expected signature is def main(**kwargs) or compatible forms. Because the function gets pickled and distributed to workers, please change global states inside the function, e.g., setting logging level, and be aware of pickling limitations. Avoid referencing large objects in the function, which might result large pickled data, making the job slow to start. kwargs – keyword arguments passed to the main function at invocation time.
Returns return value of the main function. With np>=0, this returns the value from the rank 0 process. Note that the returned value should be serializable using cloudpickle.
Releases
Visit Github Release Page to check the release notes.
License
So you have finished reading the 스파크 딥 러닝 topic article, if you find this article useful, please share it. Thank you very much. See more: 스파크 머신러닝, 스파크 완벽 가이드 PDF, Pyspark ML, Spark MLlib 예제, Spark 회귀분석, Spark .sql 한글, 아파치 스파크, Pyspark Deep learning